Programme Risk Day 2023
Friday, 15 September 2023
ETH Zurich, main building, Rämistrasse 101, auditorium HG E7
Abstracts
Roger Wattenhofer
Decentralized Finance: the Good, the Bad, and the Ugly
First, I will explain the nuts and bolts of decentralized finance (DeFi). I will put DeFi in perspective by comparing it to some adjacent concepts such as ledgers, cryptocurrencies, smart contracts, web3, etc. Then we will delve into the good aspects of DeFi, identify areas that require further improvement, and which parts of DeFi are downright ugly. In particular, I will present some spectacular attacks on the DeFi ecosystem, and what can be done to prevent such attacks in the future. Lastly, time permitting, I will explore how the underlying technology of DeFi can contribute to governance and even democracy.
Johanna Ziegel
Isotonic Distributional Regression
Isotonic distributional regression (IDR) is a nonparametric distributional regression approach under a monotonicity constraint. It has found application as a generic method for uncertainty quantification, in statistical postprocessing of weather forecasts, and it is an integral part of distributional single index models. IDR has favorable calibration and optimality properties in finite samples. Furthermore, it has an interesting population counterpart called isotonic conditional laws that generalize conditional distributions with respect to σ-algebras to conditional distributions with respect to σ-lattices. In this talk, an overview of the theory and some applications of IDR are presented.
Nora Leonardi
Let the Insurance Data Spin
Data and data-driven decisions have always been the lifeblood of the insurance industry. In recent years, data availability and technology have grown dramatically, creating new opportunities. To realise the full value, insurers can face challenges such as data silos, low data literacy, and new risks from data. Nora will talk about how we at Swiss Re got a "data flywheel" spinning, a virtuous cycle of faster, more efficient data use and smarter decisions. She will talk about tools and processes to "grease" the wheel, and share use cases and some recommendations to increase the maturity of data and analytics.
Ana Maria Matache
Using Advanced Risk Modeling to Understand the Drivers of Investment Performance
Investment performance measurement and attribution are key functions of portfolio management, bringing transparency in terms of the true drivers of portfolio risk and return. Performance analysts provide real added value and are often the only independent source equipped to understand the sources of risk and return of all portfolios and benchmarks operating within an asset management firm. Performance attribution aims to split the total outperformance of a portfolio versus its benchmark into fair contributions from the active individual decisions as well as from markets’ movements. Whereas on one hand this goal can be successfully achieved with attribution schemes that match as closely as possible the management structure of the portfolio, running different attributions on the other hand can help managers to identify unexpected, sometimes possibly unwanted sources of risk and return and adjust the management process accordingly. Performance attribution models vary from the simplest ones 1) total return or sector based attribution to the most sophisticated ones 2) factor based attribution based on a set of common risk factors and 3) hybrid performance attribution, which bridges 1) and 2). In all cases these frameworks need to accurately deal with the complexities of portfolio management: portfolio assets spread over different markets, asset classes and currencies, multiple investment horizons, multi-period compounding, derivatives handling, dynamic management, bad or missing security prices and analytics, etc.
In the first part of the presentation the focus will be on factor based attribution. Risk factor models are not only indispensable tools for risk forecasting or portfolio optimization, but also largely used for performance attribution purposes. With their choice of portfolio analytics providers investment management firms need to be very selective, since there is no such a thing like a perfect model and a proper model governance and understanding of the model strengths and weaknesses are key. We use the latest generation of risk factor model suites from Bloomberg and MSCI, both leading providers of critical decision support tools.
In the second part of the presentation we show examples on how hybrid performance attribution can be used to analyse the performance of fixed income portfolios by splitting total outperformance into two parts: that explained by bottom-up common factor aggregation and that in excess of common factors, which is further top-down (recursively) split into allocation/sector management on a user-defined hierarchical partition.
Dacheng Xiu
external page Expected Returns and Large Language Models
We extract contextualized representations of news text to predict returns using the state-of-the-art large language models in natural language processing. Unlike the traditional word-based methods, e.g., bag-of-words or word vectors, the contextualized representation captures both the syntax and semantics of text, thus providing a more comprehensive understanding of its meaning. Notably, word-based approaches are more susceptible to errors when negation words are present in news articles. Our study includes data from 16 international equity markets and news articles in 13 different languages, providing polyglot evidence of news-induced return predictability. We observe that information in newswires is incorporated into prices with an inefficient delay that aligns with the limits-to-arbitrage, yet can still be exploited in real-time trading strategies. Additionally, we find that a trading strategy that capitalizes on fresh news alerts results in even higher Sharpe ratios.
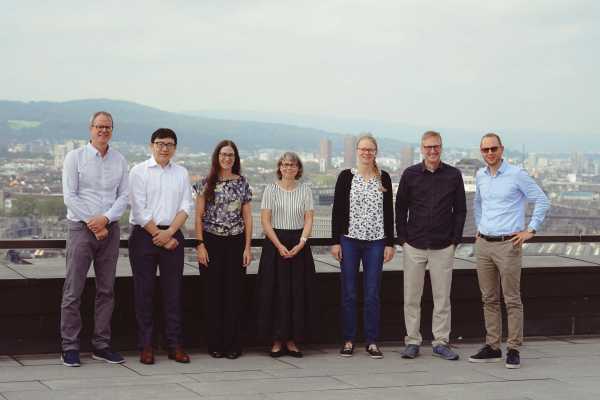
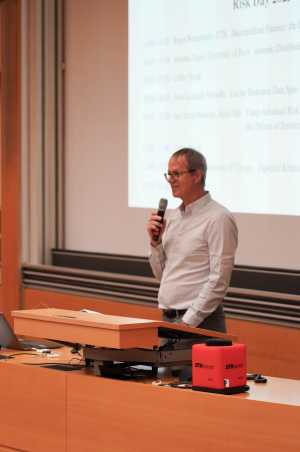
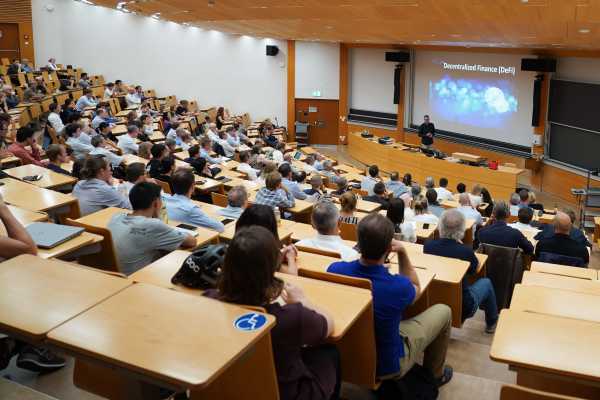
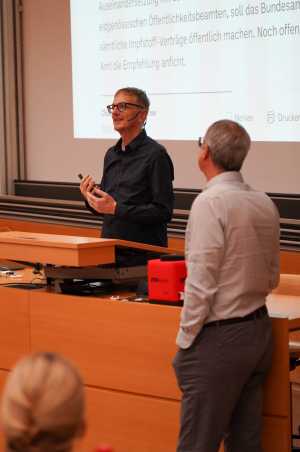
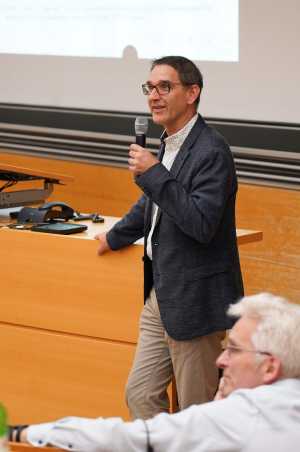
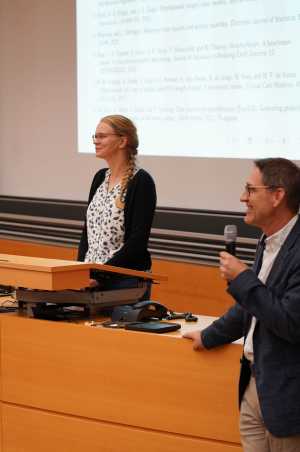
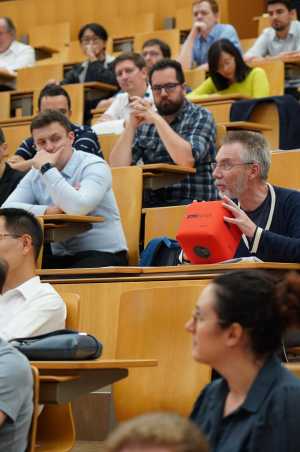
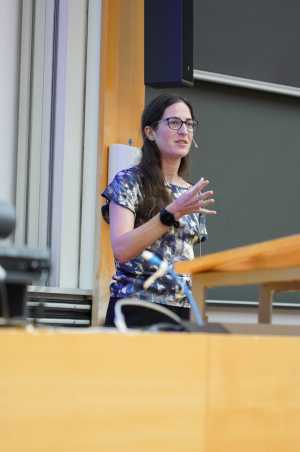
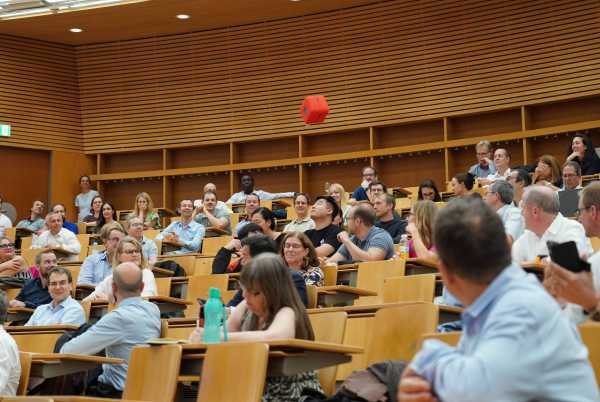
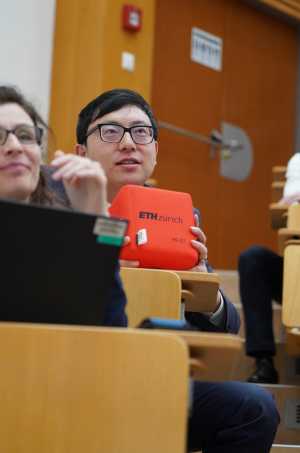
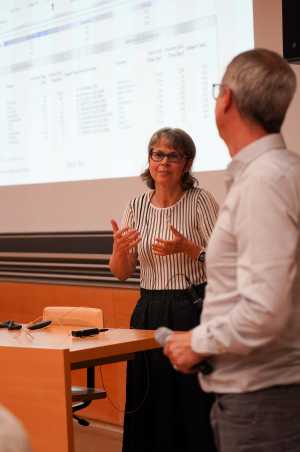
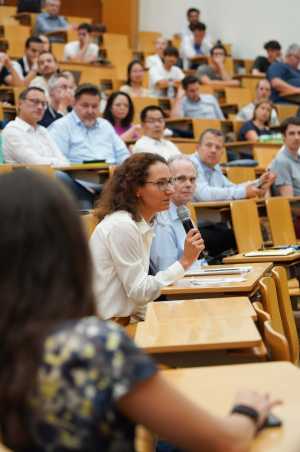
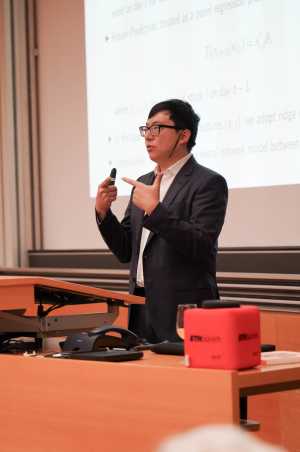